News and Stories
See news and stories about the great work we are doing at NC State to make sure our students, faculty and partners have access to the most advanced equipment, training and curricula.
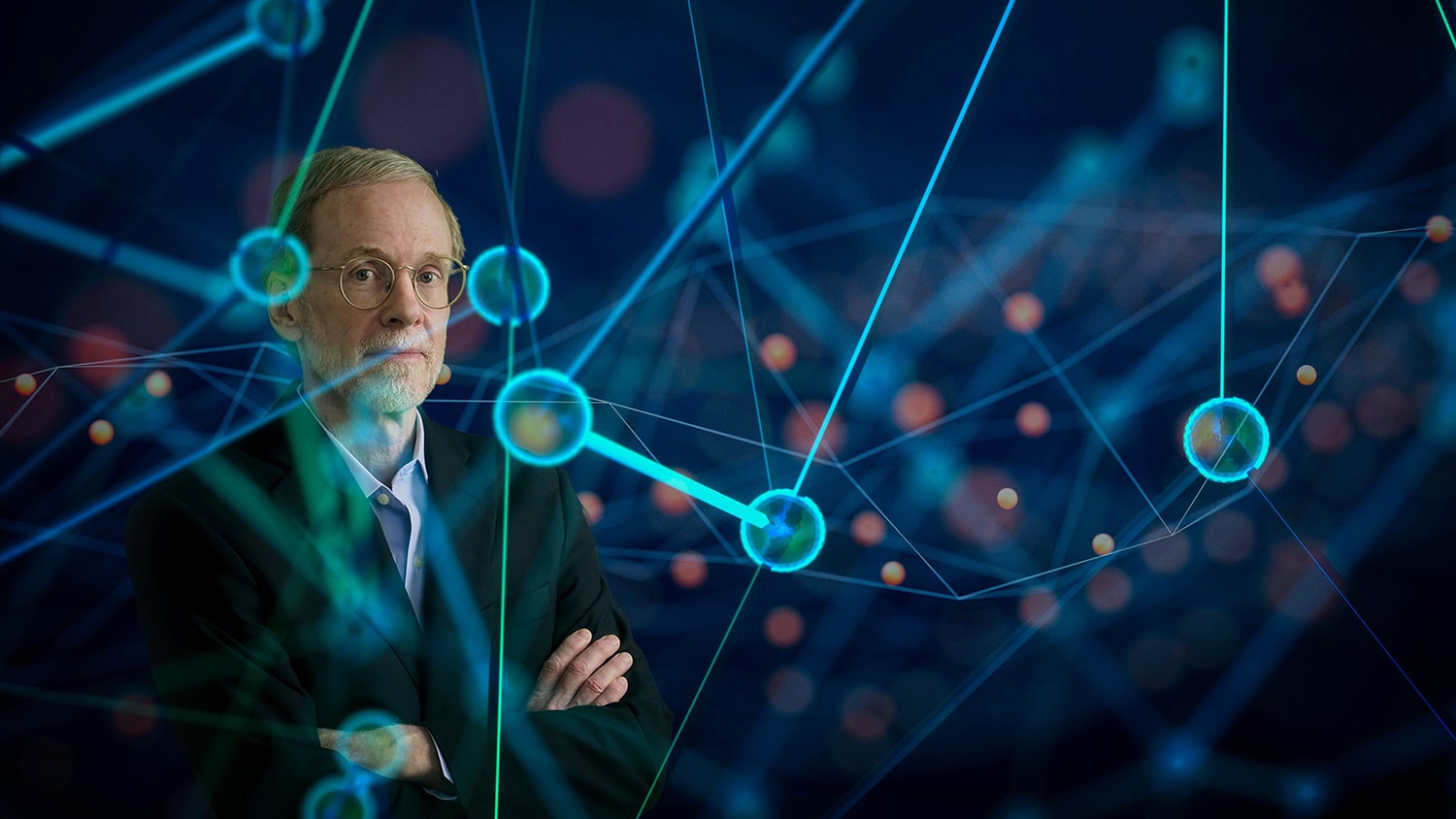
NC State, NSF Unveil Institute Focused on AI and the Future of Education
Radical innovation
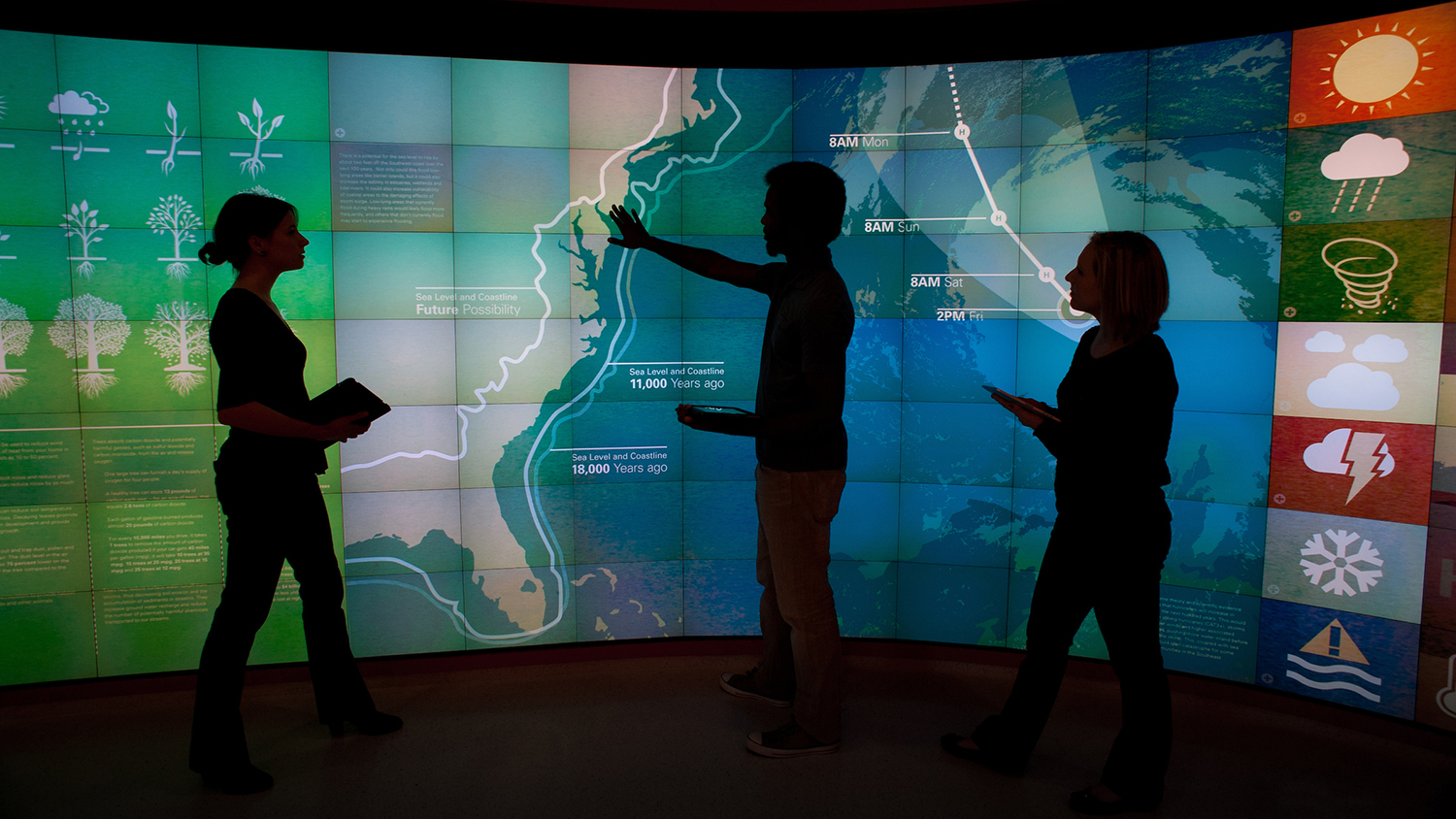
NC State Launches Universitywide Data Science Academy
Read about the academy
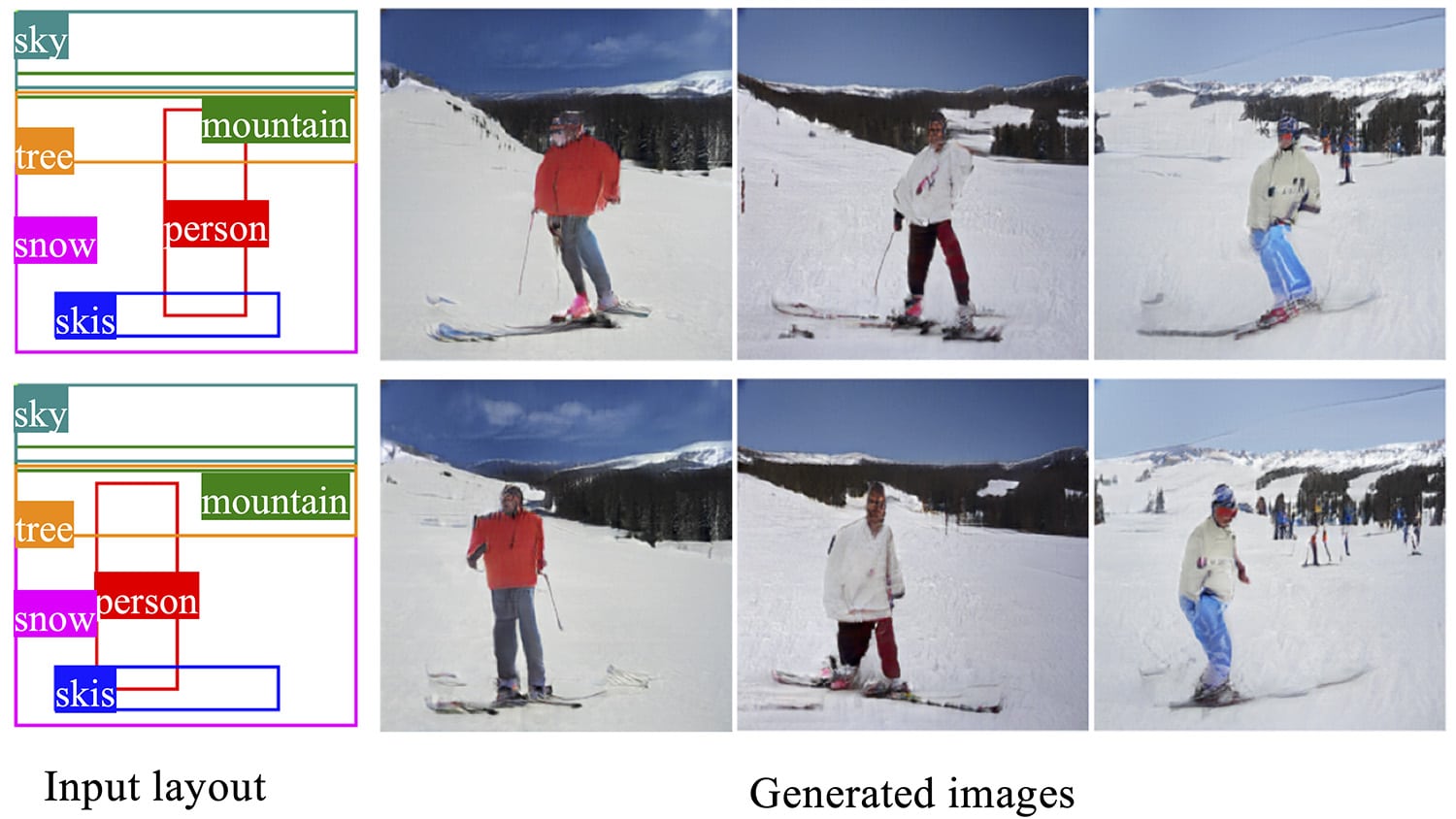
Researchers Fine-Tune Control Over AI Image Generation
Intelligent image generation
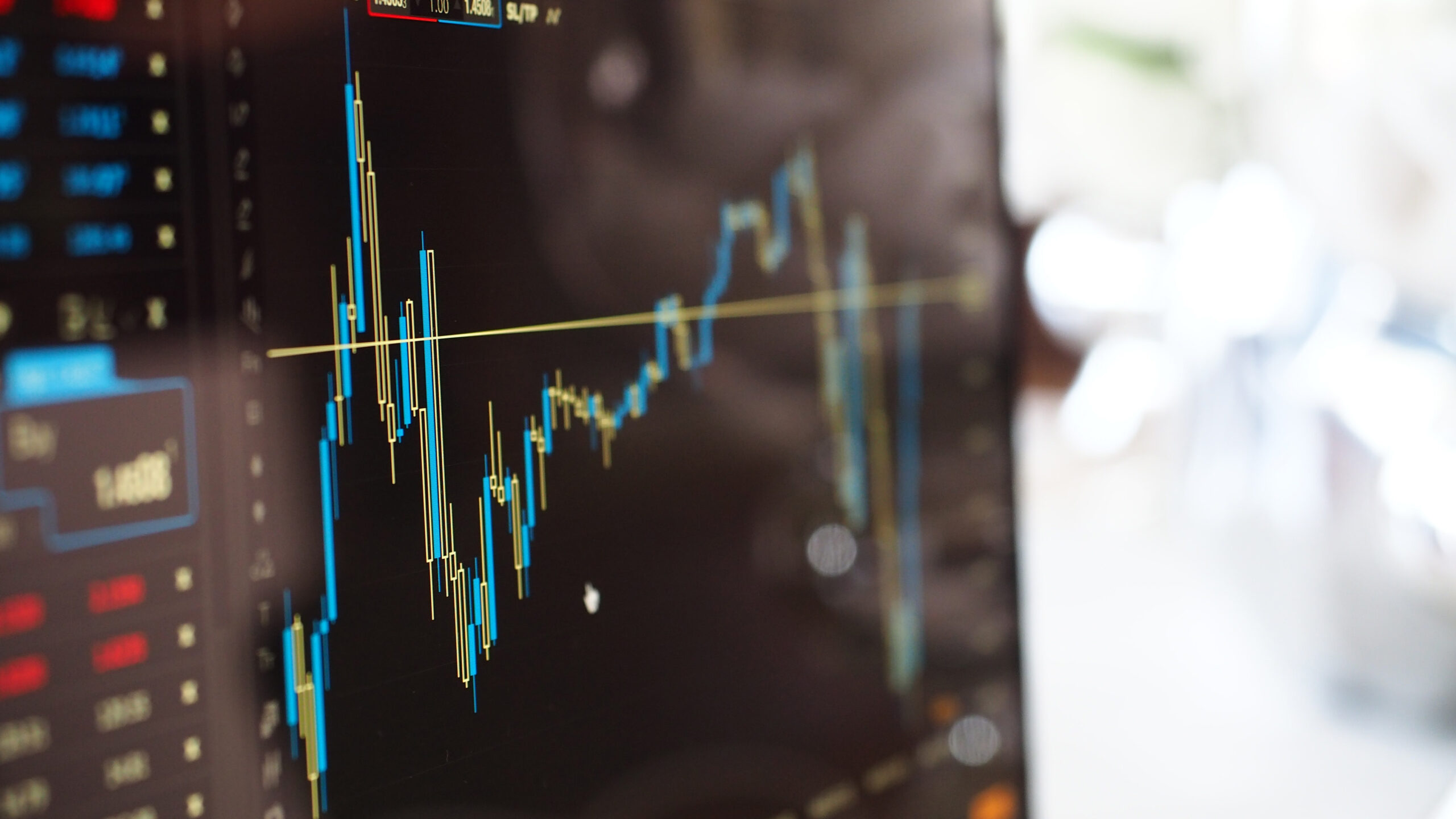
Teams of Rivals: PNNL and LAS Collaborate on Machine Learning
Read about the immersive experience
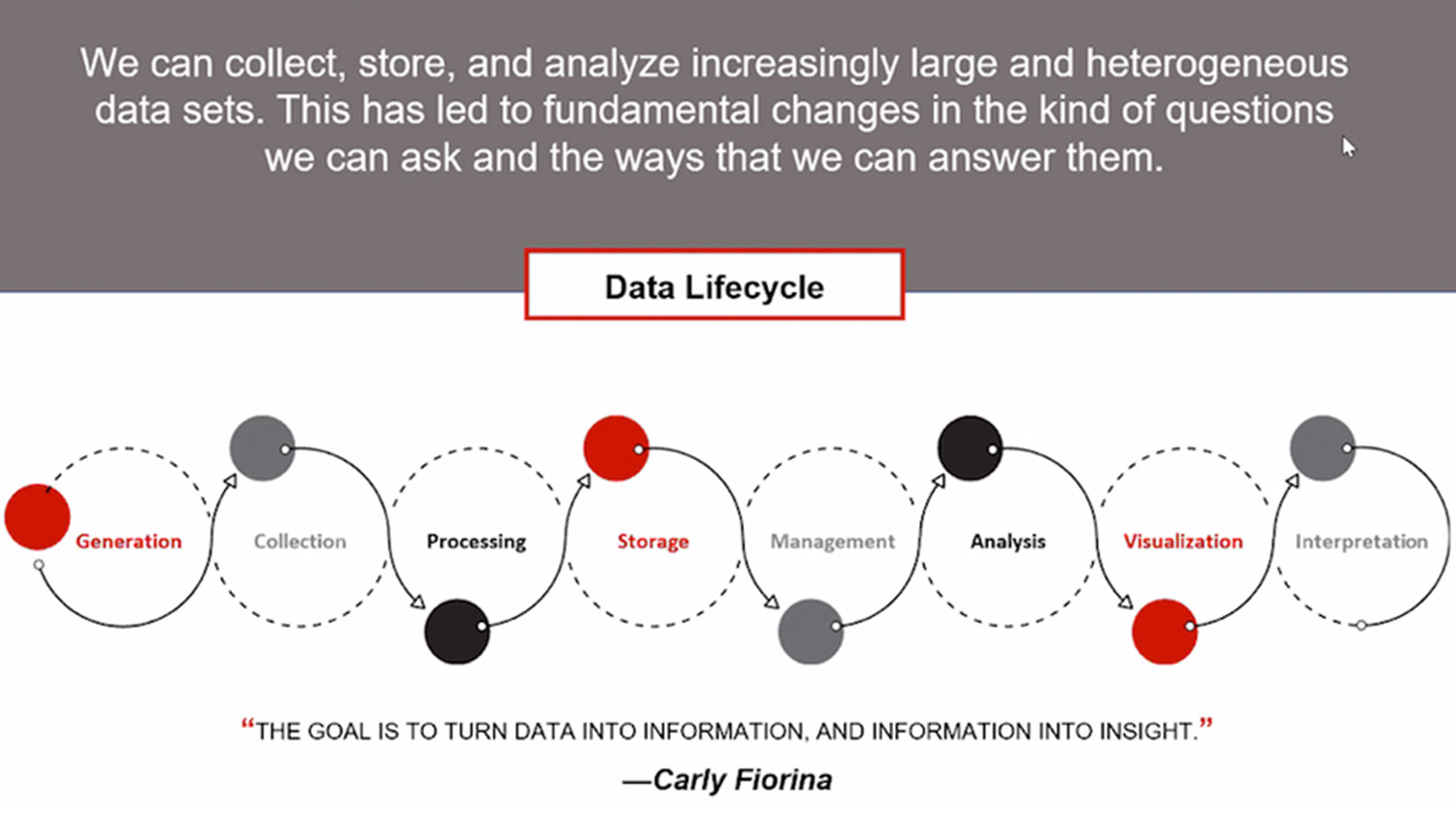
Big Data in Genetics and Toxicology
Read About How Big Data Helps Our Environment
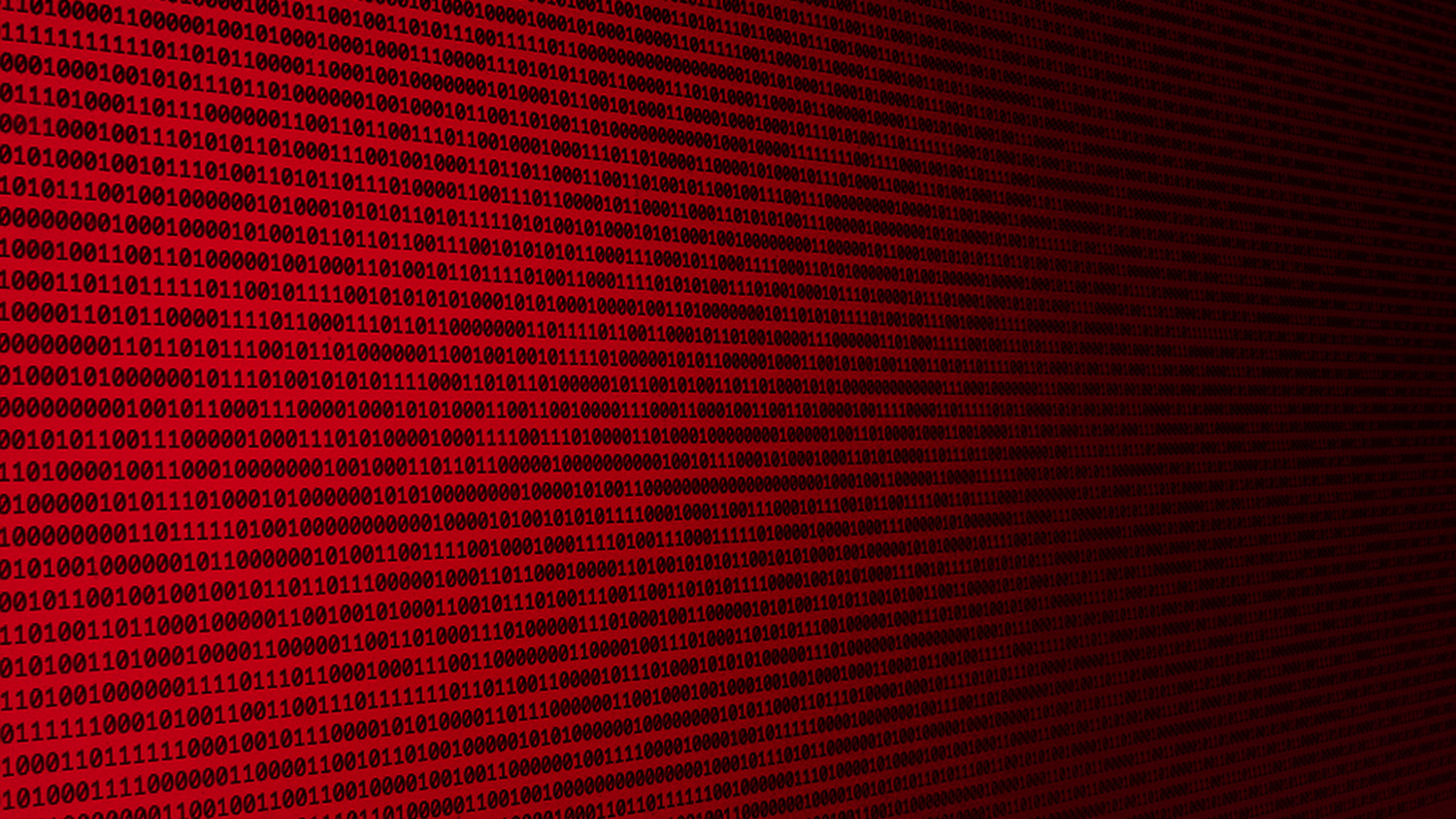
New Framework Makes AI Systems More Transparent Without Sacrificing Performance
Learn more
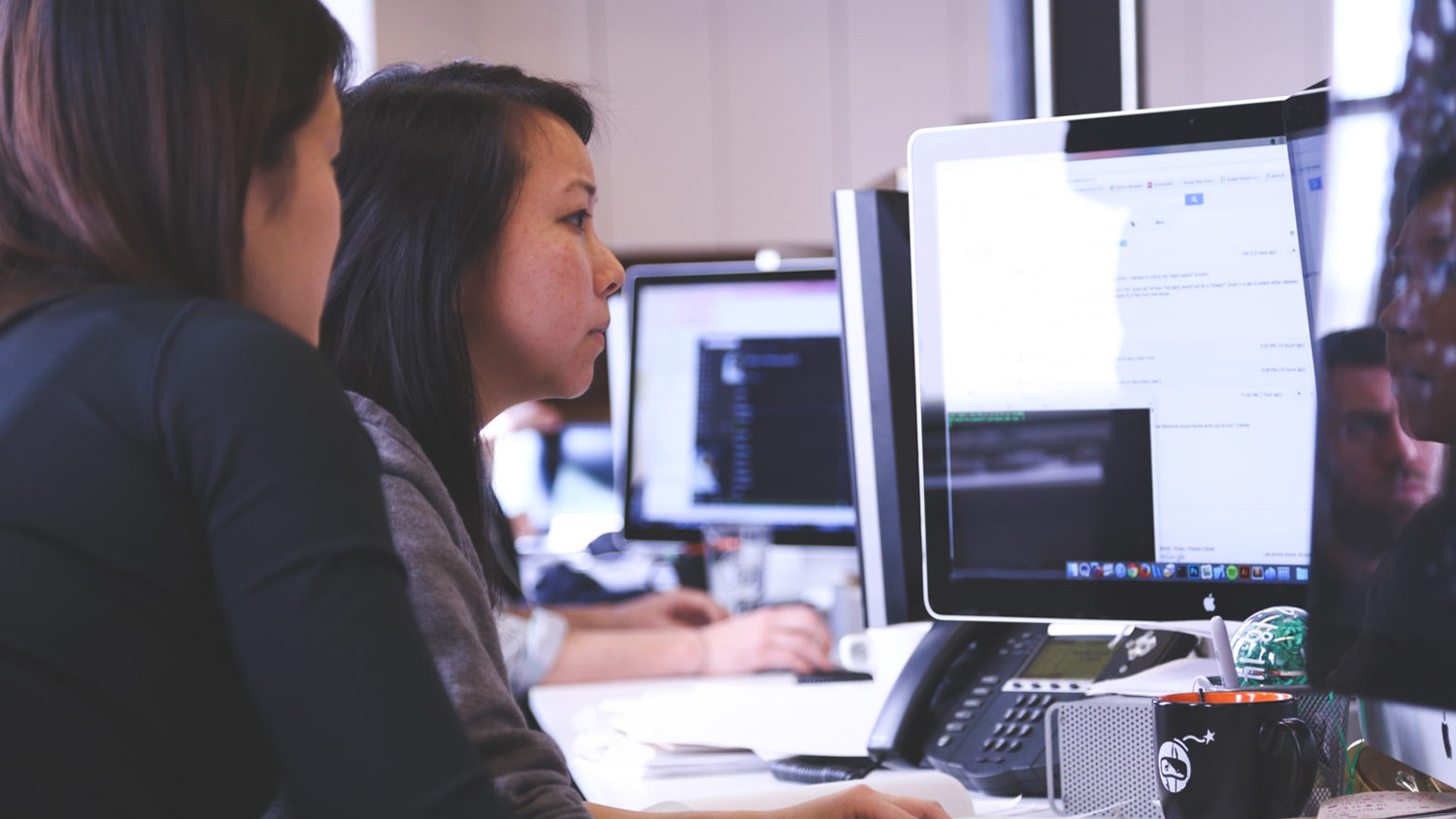
NC State and Infosys Collaborate to Develop Future Data Science Workforce
Read more
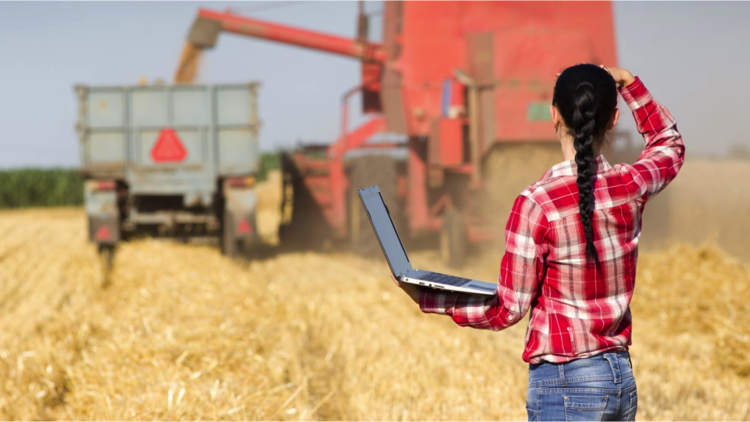
Big Data Yields Plant Sciences Innovation
Growing our data future
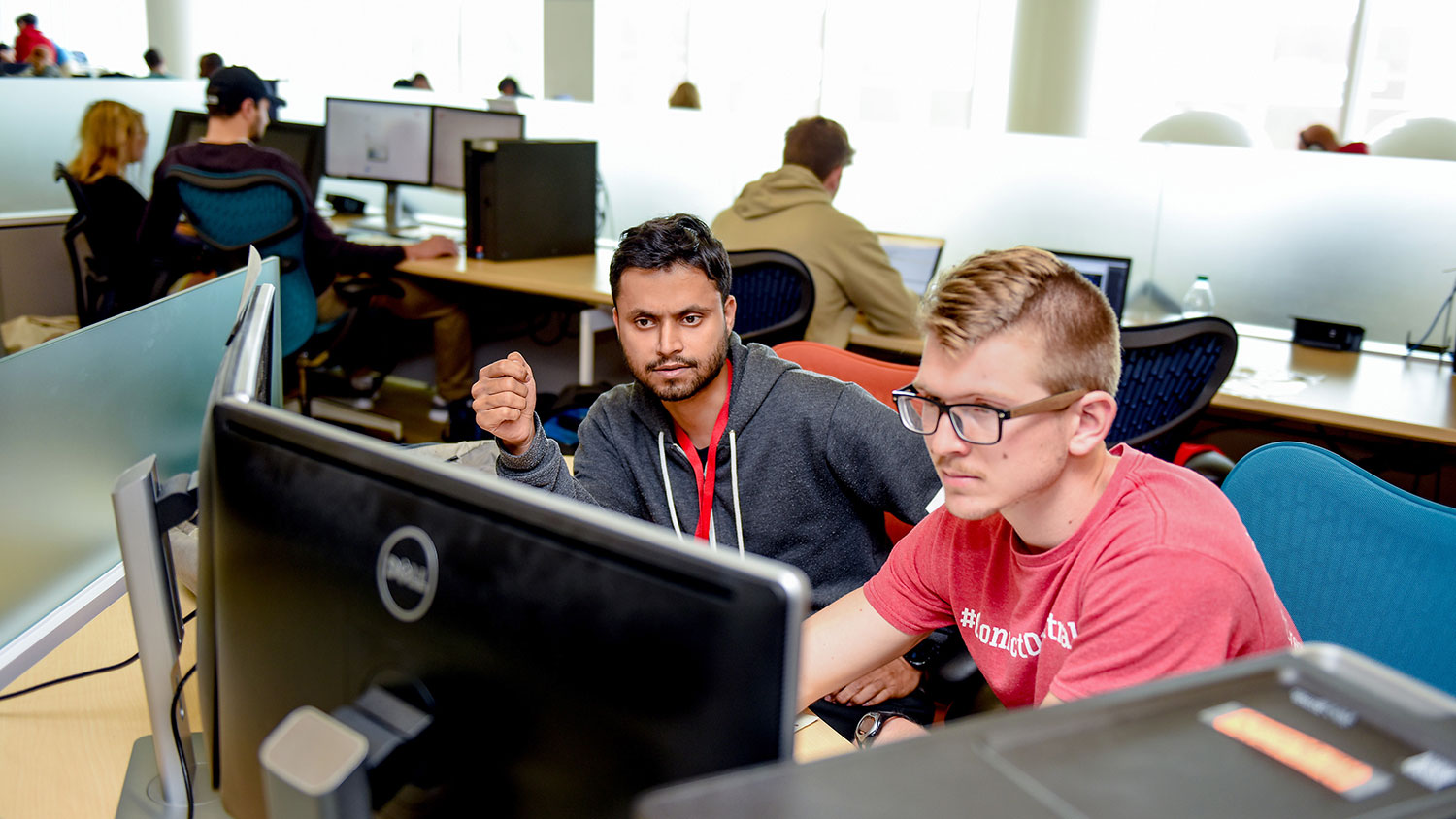
Diving Into Data
Dive in